Could AI ease food security fears?
- Like
- Digg
- Del
- Tumblr
- VKontakte
- Buffer
- Love This
- Odnoklassniki
- Meneame
- Blogger
- Amazon
- Yahoo Mail
- Gmail
- AOL
- Newsvine
- HackerNews
- Evernote
- MySpace
- Mail.ru
- Viadeo
- Line
- Comments
- Yummly
- SMS
- Viber
- Telegram
- Subscribe
- Skype
- Facebook Messenger
- Kakao
- LiveJournal
- Yammer
- Edgar
- Fintel
- Mix
- Instapaper
- Copy Link
Posted: 15 February 2021 | Dr Liangxiu Han | No comments yet
Dr Liangxiu Han discusses how we might harness big data and machine learning/AI to boost global food security in these uncertain times of climate change.
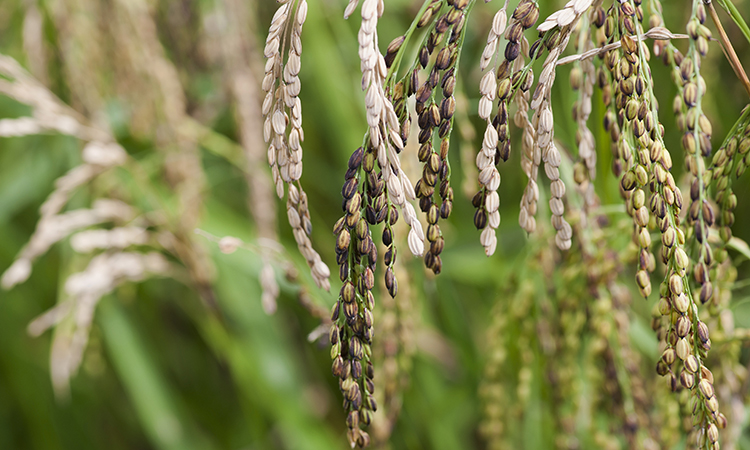
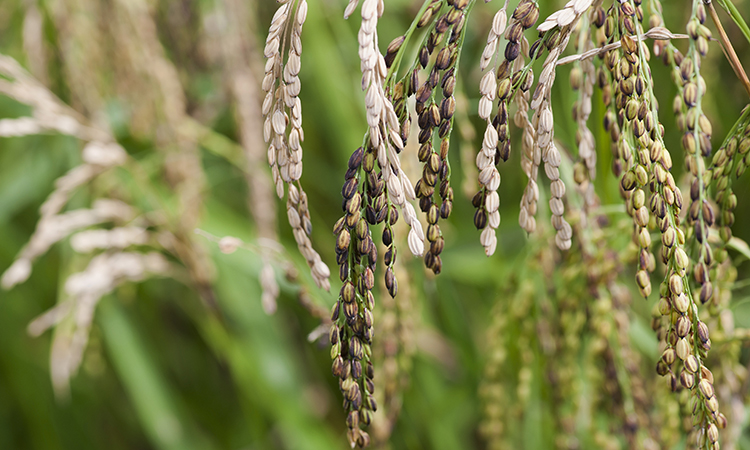
According to the UN Food and Agriculture Organization (FAO), by 2050 the projected world population is expected to be 9.1 billion; to meet this demand, global food production must increase by 70 percent.1 However, crop diseases are a major threat to food production and global food security, carrying the potential to destroy whole crops if left untreated. It is estimated that almost 40 percent of worldwide crops are lost to diseases.2
Furthermore, climate change is having a significant impact on plants, making them more susceptible to infectious diseases. It also increases the risk of spread and propagation of diseases, which could potentially lead to reductions in yield and unfavourable consequences for the environment and public health.
Crop losses tend to be the greatest in tropical countries where the environmental conditions are conducive to crop diseases. Those in less-developed countries are at greatest risk of large-scale crop losses due to lack of knowledge and inadequate technical support in effective crop disease diagnosis and control measures. Farmers use excessive amounts of chemical substances such as pesticides in an effort to protect crops from diseases; however, because they are not aware of precisely where or when to spray, this causes long‑term damage to environments and human health. In addition, disease losses can also mean more imported – and often processed – foods are eaten, replacing a balanced diet, which can create further health problems.
Early, accurate diagnosis and monitoring of crop disease occurrence and spread are crucial for precision crop management in fields, as they enable targeted interventions to address global food security. There are also cost and environmental benefits through reduced pesticide use if crops are treated during the early stages of an infestation.
Currently, diagnosis and severity assessments are performed visually by trained surveyors, which can be inaccurate, given it is subject to individual bias, as well as costly and time consuming. This is because the level of detail is variable and inevitably errors arise from the subjectivity of individuals and the tedious nature of the task. The surveyors must be regularly trained to maintain quality, which is costly. With large areas or fields to be inspected, visual inspection is very difficult, and some hotspots may go unnoticed due to only a limited number of samples being inspected. Instead, automatic, accurate and timely diagnosis and quantification of crop diseases are critical to ensure food production and sustainability.
Using AI to diagnose crop disease
Advances in big data, image processing, machine learning and artificial intelligence (AI), and cloud computing, have facilitated the development of our scalable big data-driven and AI-enabled precision agriculture solutions. This offers automated, rapid and accurate diagnosis and monitoring of crop diseases at multiple scales within and above the fields using various types of large datasets such as drones, satellites and mobile phone images.3,4,5,6 This will lead to increased farming efficiencies, reduced labour costs, and provide greater predictability for precision agricultural management.
One of our precision solutions is called AGRIONE, a machine vison system based on drone and machine learning/AI for rapid detection and mapping of rice diseases at an early stage. Images of agricultural land are captured by unmanned aerial vehicles which are then automatically analysed to identify rice plants affected by bacterial leaf blight in real time on a large scale.
By harnessing the high-resolution drone imagery and machine learning/AI, our machine vision tool is able to automatically capture subtle changes in plant chemistry and physiology caused by disease development, detecting diseases far earlier than symptoms become visible to the human eye.
Traditionally, the problem for rice farmers has been the need to diagnose the disease by visual up‑close inspection, made problematic by the difficult working conditions in the rice paddy. The areas are vast and the fields often wet, to the extent that the crop is partially submerged. In addition, once the crop has grown too tall, it is impossible to view the centre of the fields from the edges. Travelling into the fields can damage the plants, severely impacting the farmers’ ability to notice any problems. This means that disease can go unnoticed until the crop is harvested, affecting yields and profits.
By flying a drone over the fields, images can be collected and used to identify disease, if present. It can even map out its location for a targeted control response. However, most smallholder farmers do not have the money or skill to own and fly a drone in a such a way, nor do they possess the expertise to correctly identify disease in this way. AGRIONE has been developed with these limitations in mind. It is capable of diagnosing patches of diseased plants over a much larger area from the air, such as a whole rice paddy. This method is a far quicker, easier and more reliable method of diagnosing infected arable plants than by the traditional human surveyor or agronomist assessments on the ground.The AGRIONE system consists of three components: a drone survey mobile application, a farmer mobile application, and a cloud-based service platform. These three components work together to provide automatic disease detection and mapping to individual farmers.
1. The farmer mobile app: Farmers can use the farmer mobile app to request a drone survey and perform crop site-specific management for their holdings.
2. The drone survey mobile app: The drone survey mobile app can perform automated flight. The data is instantaneously transmitted back to the app and each image is automatically processed on the mobile device in real time, based on our light-weight machine learning/AI models. The user can see visual results about the location of any diseased plants on a map of the field, highlighting any infected areas in the app immediately. The results of this analysis are used to update the images, providing the user with the most accurate disease maps available at any given time. In addition, the large drone images are also uploaded to the cloud service, allowing further processing and detailed analysis.
3. The cloud service platform: The cloud service platform facilitates all the communications between the two mobile apps, hosts other app data, and provides big data processing and machine learning capability to create a detailed disease map of the farmers’ fields.
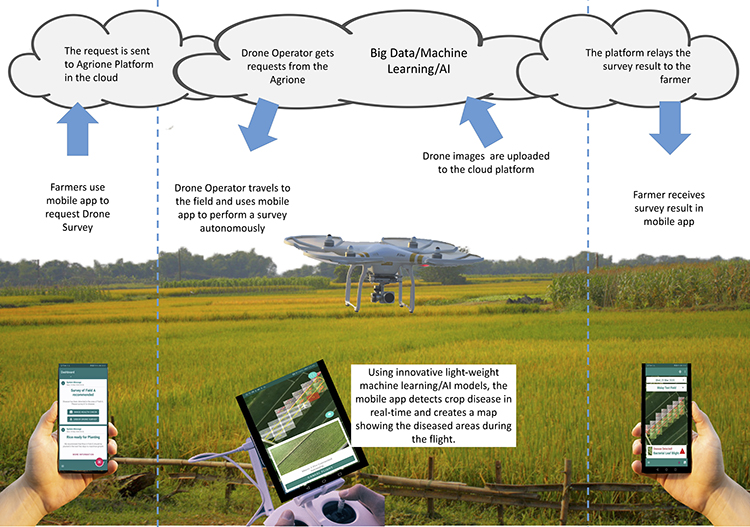
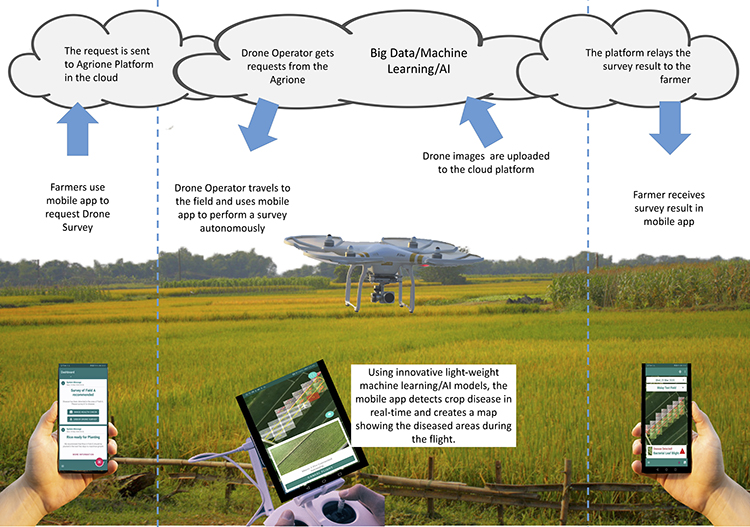
Figure 1: The high level architecture of AGRIONE
Figure 1 shows the high-level system architecture, while Figure 2 shows some example visual results of crop disease detection from the system.
Trials and commercialisation
Trials of this system are currently underway in rice paddies in Malaysia by our collaborator from University of Malaya (Co-Investigator, Dr Muhamad Shakirin Mispan) who has test fields and end users for potential adoption of our technology.
Once the technology is commercialised, it is envisaged that a farmer, smallholder or agricultural collective could buy, or more likely hire, the equipment from a third-party supplier to conduct surveys. Another approach could be that government agency outreach workers provide or loan the equipment to farmers as part of a wider agricultural support package.
Approximately 400,000 small farmers in Malaysia depend on rice cultivation and these farmers can make immediate use of our solution to increase productivity and reduce cost. Public sector organisations, such the Malaysian Agricultural Research and Development Institute and Malaysia’s Department of Agriculture, can use our solution to provide better services for farmers. In addition, the successful adoption of our technology will help shape regulation and government policies in relation to data-driven, AI-enabled smart agriculture in Malaysia.
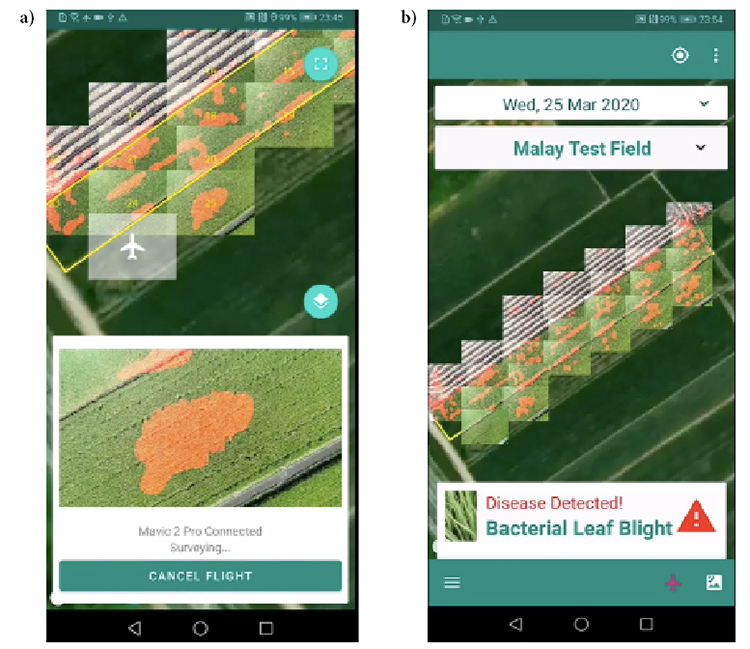
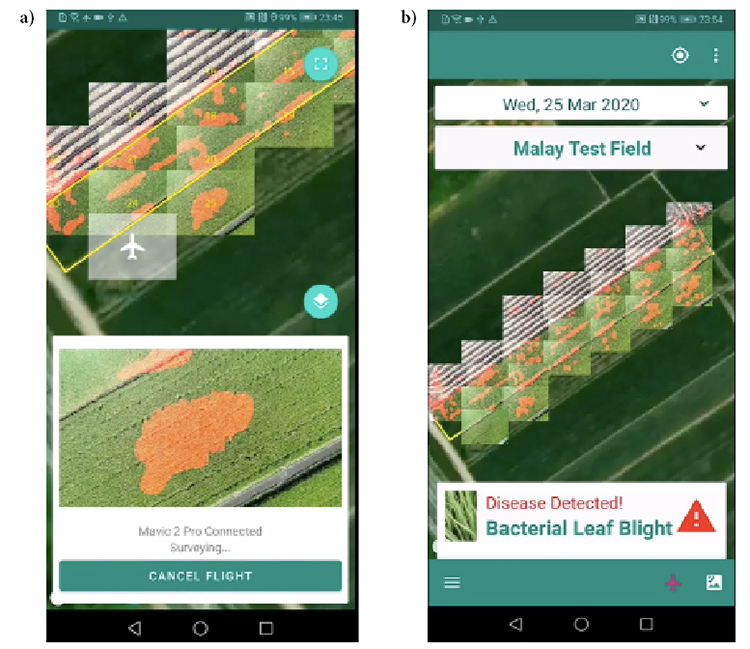
Figure 2: Visual results of drone survey and crop disease detection
a) Automated survey and crop disease detection in real time during the flight
b) Visual result of disease mapping after the flight
Once our technology is rolled out, our plan is to deploy it to other developing countries where resources and technical support are limited. In addition, we will augment our system with a spraying function for effective disease and weed control, helping to maintain crop health and yield (eg, spraying pesticides at the right time and in the right location).
Although we are working with rice fields and bacterial leaf blight, our general modular-based platform is scalable and can process satellite images, drone images and other datasets that can be adapted to other crops and applications; for example, weed and soil health assessment or irrigation.
References
- FAO, Global agriculture towards 2050, http://www.fao.org/fileadmin/templates/wsfs/docs/Issues_papers/HLEF2050_Global_Agriculture.pdf
- Strange R: Food security 2(2), 111 (2010). doi:10.1007/s12571-010-0064-5
- Zhang X, Han L, et al. “A Deep Learning-Based Approach for Automated Yellow Rust Disease Detection from
High-Resolution Hyperspectral UAV Images”, Remote Sen. 2019, 11(13) - Ma H, Huang W, Jing Y, Yang C, Han L, et al.
“Integrating Growth and Environmental Parameters to Discriminate Powdery Mildew and Aphid of Winter Wheat Using Bi-Temporal Landsat-8 Imagery”, Remote Sensing, 2019, 11(7), 846. - EPIC: An automated diagnostic tool for Potato Late Blight disease detection from images, https://epic337255300.wordpress.com
- AGRIONE: http://agrione.farm
About the author
Dr Liangxiu Han is a Professor of Computer Science, Co-Director of the Centre for Advanced Computational Science and Deputy Director of the Big Data Centre at Manchester Metropolitan University.
Having worked in both academia and industry, Prof Han has extensive research and practical experience in developing big data-driven, machine learning/AI-enabled intelligent solutions in different application domains (eg, precision agriculture and health) using various types of big data (eg, images, sensors, videos, text, etc) to address societal challenges.
Issue
Related topics
Data & Automation, Equipment, Food Safety, Food Security, New product development (NPD), Quality analysis & quality control (QA/QC), Rapid Detection, Research & development, Robotics & automation, Supply chain, Sustainability, Technology & Innovation
Related organisations
Food and Agricultural Organization of the United Nations (FAO), Manchester Metropolitan University, University of Malaya