Understanding the capabilities of your lab method
- Like
- Digg
- Del
- Tumblr
- VKontakte
- Buffer
- Love This
- Odnoklassniki
- Meneame
- Blogger
- Amazon
- Yahoo Mail
- Gmail
- AOL
- Newsvine
- HackerNews
- Evernote
- MySpace
- Mail.ru
- Viadeo
- Line
- Comments
- Yummly
- SMS
- Viber
- Telegram
- Subscribe
- Skype
- Facebook Messenger
- Kakao
- LiveJournal
- Yammer
- Edgar
- Fintel
- Mix
- Instapaper
- Copy Link
Posted: 18 February 2021 | Erik Konings, Fabien Robert | No comments yet
Two Nestlé experts outline important information around fit-for-purpose methods within analytical chemistry and what it means for the food manufacturer.
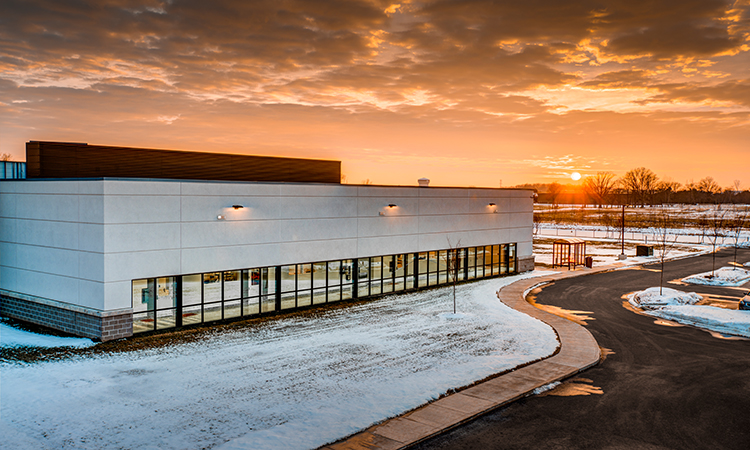
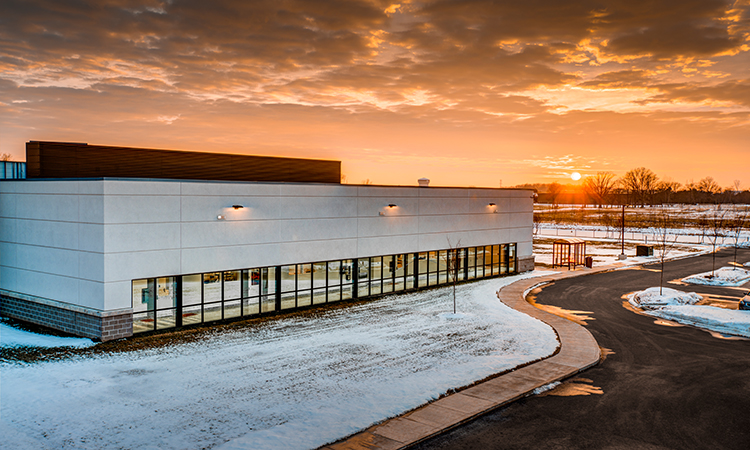
Fitness for purpose of an analytical method is a well established concept in the scientific community. However, it tends to rely on analytical performance characteristics to provide an answer to specific requirements. Precision is usually one of the requirements and is often set based on technical experience1 rather than what is required in the frame of regulatory and/or production limits.
It is important to understand the capability of a method; ie, its ability to produce test results within the regulatory/production limits. The validation of the analytical method gives an indication of result variation generated by the method. It is therefore an important part of assessing the fitness for purpose, but we should not underestimate the significance and impact of other sources of variability, such as raw material and the production process.
In this article, we review the key performance characteristics of analytical methods, the process capability concept and its link with method variability performance characteristics. Finally, we will discuss the holistic approach that can be taken to ensure adequate fitness for purpose for the analytical method employed. This understanding is critical for food manufacturers to ensure full product compliance.
Analytical method performance criteria and method variability
Analytical method performance characteristics commonly evaluated during method validation encompasses applicability, limit of detection (LOD) and limit of quantification (LOQ), working range, trueness (bias and recovery), precision (repeatability, intermediate precision and reproducibility), ruggedness, and, to a certain extent, measurement uncertainty. The latter is not exactly a performance characteristic of a method, but often constitutes an important element.
While LOD and LOQ are relatively simple characteristics to assess against a given requirement, overall analytical method precision is more complex as it is subject to some intrinsic and extrinsic parameters.
To establish whether LOD and LOQ is fit-for-purpose, they must be checked against a regulatory requirement or health guidance value,2 but generally, they do not trigger major challenges. However, it is important to understand the purpose of the requirement and how these values will be used as lower bond, mid bond or upper bond, for example, in dataset treatment.
From an analytical viewpoint only, LOQ can be used to report a precise number. Ruggedness of a method is also an important element as it indicates how the method can be stretched in terms of scope of use.
Process capability and analytical method capability
Six Sigma is an initiative for continuous improvement, developed by Motorola engineer Bill Smith in the mid-1980s.3 In this approach, sigma (σ), used by statisticians to denote SD (standard deviation), can be considered as process variability,4 a concept representing the amount of variability relative to requirements or specifications. From there, the process capability index (Cp) can be defined as a measure of the ability of a process to produce results that meet specifications.5 It represents the ratio between the acceptable spread (specifications) of results and the actual spread (process variability). The process capability index compares the specifications for a given parameter to six times the estimated process variability (SDp).
Process capability Cp =
product specification range/6x SDp
Assuming the results are normally distributed and the product is manufactured on target, it is possible to derive a direct link between the Cp and expected percentage of products out of specification. For example, if a parameter (nutrient) in a production process has a Cp of one, it is expected that 0.27 percent of the products will be out of specification for the parameter (nutrient) of interest based on 99 percent confidence level. Applying the same principle, the impact of analytical method variability on the assessment of product compliance can be assessed for a given analytical method by defining a method capability index (Cm), determining the extent to which a method can demonstrate compliance.6 The method capability can be defined as: ‘method capability Cm = product specification range/6 SDm’ where SDm is the method standard deviation.
Assuming normal distribution, a direct link can be derived between the Cm value and expected percentage of values out of specifications due to method variability, in much the same way as Cp.
The relationship between specification range, process variability and analytical variability is illustrated in Figure 1.
Since the method variability is included in the overall process variability SDm<SDp, and consequently, Cm>Cp, the requirement for Cm to achieve the required Cp directly depends on the weight of the method variance within the overall variability as ‘Cm = Cp × (1/w)1/2’ where ‘w’ represents the proportion of total variance attributable to measurement variance.
The variance of an analytical method is chosen arbitrarily to be ‘w = 1/3’ of the overall process variance, where the other 2/3 are considered to come from ingredient and manufacturing process variability, respectively. Consequently, a Cm should be at least 1.73Cp. In that case, a Cm < 1 would be critical.
By considering a weight of the method variance equal to 1/3, any method with Cm < 1.73 would be problematic for achieving a Cp of one (0.27 percent products out of specification range) and a Cm < 1 would be critical. It should be noted that the 1/3 factor can vary depending on analytical method/analyte, matrix and production process.
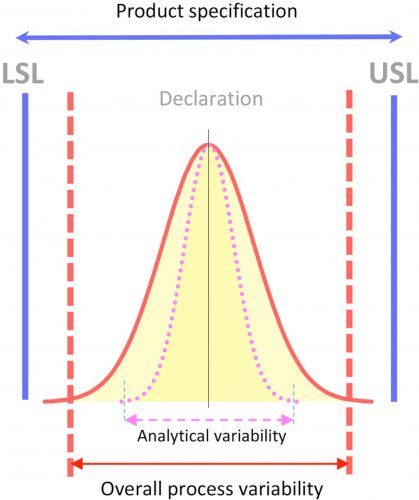
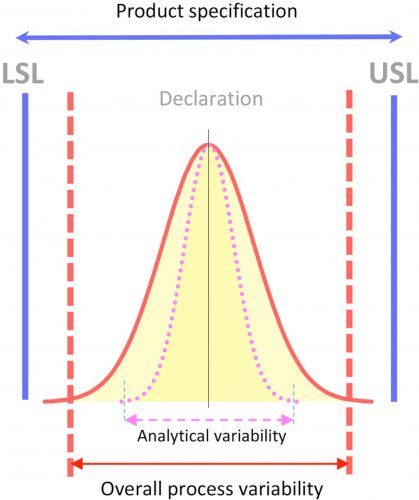
Figure 1 Product specification and variability. LSL = lower specification limit.
USL = upper specification limit
Discussion
While analytical method performance characteristics are essential in the fitness for purpose of a method against a set of requirements being internally defined or regulatorily mandated, it is of utmost importance to understand the overall variability in the system observed, including process and raw material.
As expressed in Figure 1, the analytical variability should be embedded into the overall possible variabilities, which should be within the LSL and the USL for each requirement.
The concept of method capability can assist in setting performance requirements of an analytical method. A target precision for an analytical method can be designated based on a required Cm and specification limits, taking into consideration the variability of ingredient sources and the production process.
Without a proper assessment and deep understanding of the process capability, the risk of wrongly attributing the overall variability on the analytical method is more likely. This leads to an analytical method that does not look fit for purpose in practice, while the validation file may appear as fit for purpose. The consequence may be an incomprehension between the analytical results from the laboratory and the manufacturing stakeholders who may challenge the analytical result without having necessarily integrated the other root causes of variability.
To avoid this situation, the development of new manufactured food must encompass the proper quality by design and master the processing parameters linked to processing critical control points. Thus, for each requirement defined in the HACCP plan and in the product specification requirements, it is essential to:
- Have good control over the raw material variability
- Master the processing parameters that are affecting process capability on the defined requirements you want to have in your final product
- Properly define the process capability through adequate validation of the design
- Engage with analytical method development partners as early as possible to define the analytical method capability and the link between the Cm and the Cp
- Integrate Cm and Cp values in your release criteria and in the performance criteria of the analytical method before routine production.
To summarise, the overall variability on a manufactured product can schematically have three main root causes. The variability can come from the raw materials, the processing conditions, and the analytical method used to measure the desired control point. These three factors are too often assimilated to the unique measure of uncertainty of the analytical methods, while the raw material capability and the process capability are neglected and sometimes not well understood. To avoid challenges while in production mode, quality by design during the development phase is key. Mastering your processing in conjunction with your analytical method capabilities will demonstrate compliance to your given set of requirements, whether that is internal specifications or regulatory framework.
References
- Horwitz W, Kamps LR, Boyer RW. “Quality Assurance in the Analysis of Foods for Trace Constituents.” J. Assoc. Off. Anal. Chem., Vol. 63, No. 6, 1980; pp. 1344- 1354.
- Konings E.J.M., Roux A., Reungoat A. et al. Challenge to evaluate regulatory compliance for nutrients in infant formulas with current state-of-the-art analytical reference methods. Food Control 119 (2021) 107423
- Snee RD. (2010). Lean Six Sigma – Getting better all the time. International Journal of Lean Six Sigma, 1 (1), pp. 9-29
- Pyzdek T, Keller PA. (2010). The Six Sigma Handbook: A Complete Guide for Green Belts, Black Belts, and
Managers at All Levels. 3rd Edition, McGraw-Hill, New York. pp. 140-142, 252-273, 295-300, 377-385, 452-464, 595-599. - Burnett L. Application of process capability indices to quality control in a clinical chemistry laboratory. Clinical Chemistry, Vol. 42, issue 12 December 1996.
- Bais R. (2008). Use of capability index to improve laboratory Analytical Performance. Clin Biochem Rev: 29(Supple1), S27-S31.
About the authors
Fabien Robert
Erik Konings
Issue
Related topics
Equipment, Food Safety, Quality analysis & quality control (QA/QC), Research & development, Sensory technology, Supply chain, Technology & Innovation