The pros and cons of unified data for R&D
- Like
- Digg
- Del
- Tumblr
- VKontakte
- Buffer
- Love This
- Odnoklassniki
- Meneame
- Blogger
- Amazon
- Yahoo Mail
- Gmail
- AOL
- Newsvine
- HackerNews
- Evernote
- MySpace
- Mail.ru
- Viadeo
- Line
- Comments
- Yummly
- SMS
- Viber
- Telegram
- Subscribe
- Skype
- Facebook Messenger
- Kakao
- LiveJournal
- Yammer
- Edgar
- Fintel
- Mix
- Instapaper
- Copy Link
Posted: 9 December 2021 | Noel Hollingsworth | No comments yet
Uncountable’s CEO, Noel Hollingsworth, explains the process behind unifying data and what the benefits and pitfalls may include.
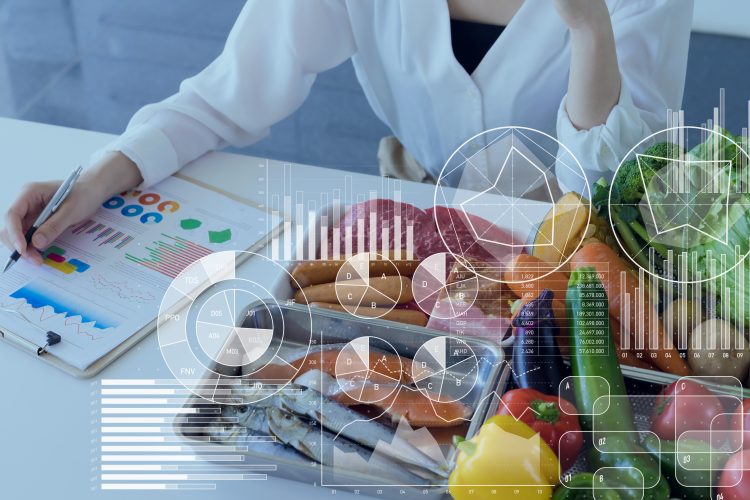
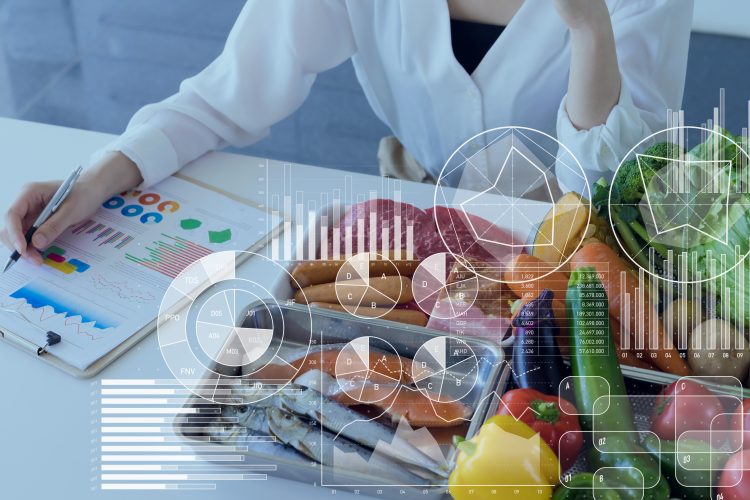
As R&D teams seek to digitalise their workflows, they encounter the same recurring theme – a proliferation of data from numerous sources, and multiple standards and processes in place. Where previously digitalisation focused on making data available in a computerised form, now companies need to make data usable without friction – either by scientists or by computers, ie, artificial intelligence (AI). Unifying different data sources is typically a necessary step for usability and promises an excellent ROI – but it isn’t cheap. This article outlines the process of unifying data, highlighting the benefits and downsides.
Unified data
The goal of a unified data system is to unite different data sources in a single system. An example of an ununified system might be an ELN that holds experimental notes, a LIMS system that records the results of developmental and production trials, another system for recording consumer data, and yet another for regulatory data. It may be easy to find data in one of these systems, but it is often very time consuming to tie the data across systems and understand how an experimental change recorded in the ELN led to a different observed output behaviour in the LIMS, which led to different customer data.
The process of unifying the data typically works by either implementing a more complete system that replaces multiple existing systems, or by trying to link the data within a hub system. A mixed approach often works best; it is common for ELN + LIMS to be replaced with a modern solution that brings the best of both frameworks, but pricing information is often still pulled from a production ERP system.
Downsides of unified data
The two primary downsides of the unified approach are switching costs and the need for more rigorous data entry. The first cost is more obvious – there are existing systems in place that scientists are used to working with and that IT has vetted. A unified approach requires leaving some of these systems and tying others into the new framework. Change management will be needed from both R&D and IT. Organisations must ensure that any new provider will work with them and be invested in the success of this changeover. It would be a costly mistake to pay a software provider a large upfront fee and not work with them afterwards, as this disincentivises the original vendor from ensuring success. Modern approaches like subscription-based billing help align incentives here.
The less obvious downside is the need for rigour in a unified system. When every scientist works in a lab notebook page, it is acceptable for one scientist to call something “Ing A”, another “Ingredient A”, and another “Trade Name XYZ”. However, the entire benefit of a unified system is standardising this information. The organisation should clarify internally that the goal is to represent each object in a consistent manner, ensuring that the provider in question can accommodate this. Features such as access control rights for who can edit inputs/outputs and merging of data should be easily achievable.
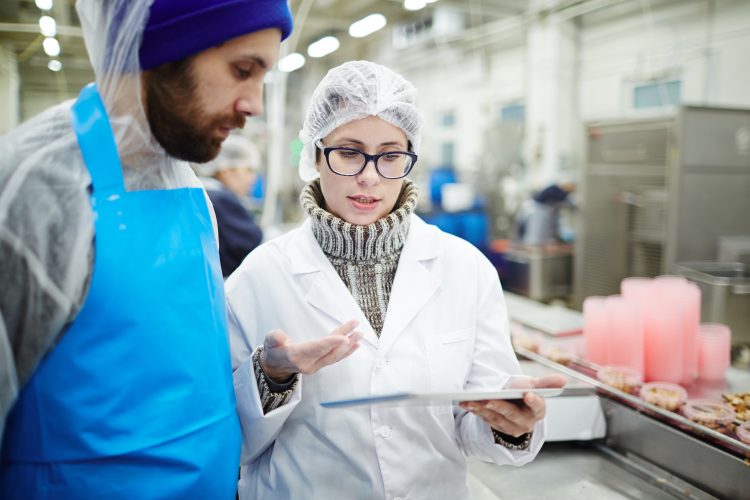
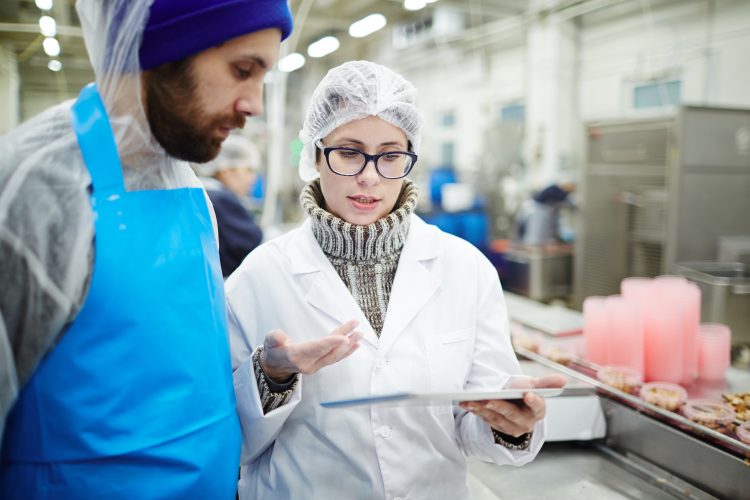
Unified data can make data easier to find – especially for bigger teams
Benefits of unified data
Despite the concerns of unifying data, the primary benefits can provide a high ROI, especially for organisations with larger teams. The first key benefit is simply the ease of finding data. Being able to find a formulation used in an R&D (not production) experiment that met certain output targets and uses certain ingredients often takes a phone call today. When that becomes a 15-second query, scientists can spend more time innovating and less time cleaning data.
Once data can be found easily the next step is ease of visualisation and analytical capabilities. Many scientists within R&D organisations work by extracting data from these systems, cleaning it in Excel, and then plotting it in a standalone programme. Each step of this process takes time and creates the possibility of introducing errors. Most R&D teams have questions they would like to ask of their data but have never got around to because simply compiling the information would take too long. These questions can be answered far more easily with a simple querying and filtering process in one place, rather than reaching across multiple programmes.
The end goal for many teams of a unified system is AI capabilities. While it is necessary to caution that wins using AI will not be immediate, AI can be a major benefit of a unified data approach. AI software needs clean data to work – no algorithm can rectify inputs and outputs not being jointly available, or input label inconsistency. Today, because data is split across multiple systems, AI work often involves one-off projects with lengthy data cleaning phases; these introduce potential for error and shrink the available data pool. A unified system will help ensure that AI work is part of a standard workflow, enabling it to be used more often across an organisation.
Conclusion
Moving to a unified data system requires both effort and attention to rigour within a business. But if organisations accept this, there are many benefits to be reaped – from searching through the data, analysing the information and eventually AI capabilities as well.
About the author
Noel Hollingsworth is the CEO and one of the Co-Founders of Uncountable, which works to centralise scientific development data across a number of industries, including cosmetics, personal care, flavours and fragrances, and more. Noel has a background in software engineering, and prior to his work at Uncountable was named to Forbes 30 under 30 for his work in machine learning. Today, Noel works directly with Uncountable’s customers to implement their vision for a centralised data platform, helping them to innovate quicker to meet modern consumers’ ever changing needs.