Food adulteration detection and measurement with NIR hyperspectral imaging
- Like
- Digg
- Del
- Tumblr
- VKontakte
- Buffer
- Love This
- Odnoklassniki
- Meneame
- Blogger
- Amazon
- Yahoo Mail
- Gmail
- AOL
- Newsvine
- HackerNews
- Evernote
- MySpace
- Mail.ru
- Viadeo
- Line
- Comments
- Yummly
- SMS
- Viber
- Telegram
- Subscribe
- Skype
- Facebook Messenger
- Kakao
- LiveJournal
- Yammer
- Edgar
- Fintel
- Mix
- Instapaper
- Copy Link
Posted: 22 February 2016 | Marena Manley, Professor, Stellenbosch University / Federico Marini, University Researcher, University of Rome ‘La Sapienza’ | No comments yet
Food adulteration, e.g. adulteration of spices, can be incidental or intentional. Incidental adulteration occurs when foreign substances are added to a food due to ignorance, negligence or using inadequate facilities. Intentional adulteration, also referred to as economic adulteration, entails the deliberate addition of inferior materials to a food to improve appearance qualities and value for economical gain. Nevertheless, adulteration of food products may lead to legal, medical or religious disputes. Over the years several analytical methods have been considered to reliably detect adulterants in food products. Vibrational spectroscopy has received particular attention due to it being environmentally-friendly, not requiring sample preparation and providing immediate results. One of the advantages of performing spectroscopy measurements in the near-infrared (NIR) compared to the mid-infrared (MIR) region is its ability to penetrate deeper into the sample and to measure larger areas or volumes . Although the sample area analysed with NIR spectroscopy is much larger than in the case of MIR spectroscopy, the results are still reported as an average measurement. NIR hyperspectral imaging, which represents the new frontier of spectroscopic techniques, advances on conventional (or bulk) NIR spectroscopy by providing an additional spatial dimension.
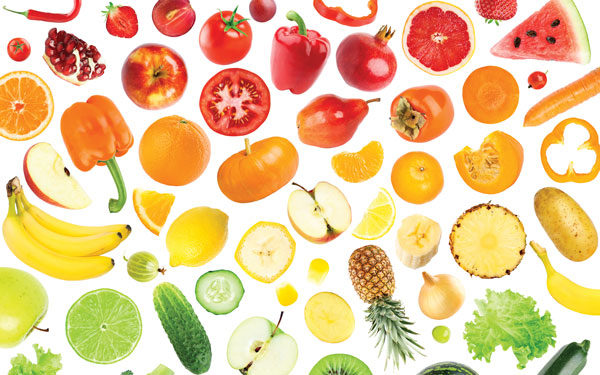
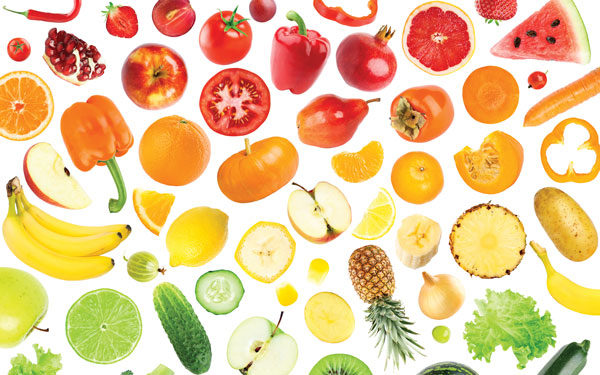
Over the years several analytical methods have been considered to reliably detect adulterants in food products. Vibrational spectroscopy has received particular attention due to it being environmentally-friendly, not requiring sample preparation and providing immediate results. One of the advantages of performing spectroscopy measurements in the near-infrared (NIR) compared to the mid-infrared (MIR) region is its ability to penetrate deeper into the sample and to measure larger areas or volumes1 . Although the sample area analysed with NIR spectroscopy is much larger than in the case of MIR spectroscopy, the results are still reported as an average measurement. NIR hyperspectral imaging, which represents the new frontier of spectroscopic techniques, advances on conventional (or bulk) NIR spectroscopy by providing an additional spatial dimension1 .
NIR hyperspectral imaging is similar to conventional colour photography, where for each basic unit of the image (i.e. a pixel) the levels of green, red and blue are recorded; the only difference being that, instead of only three spectral intensities, an entire profile is collected for each pixel or spatial point. NIR hyperspectral imaging is different to RGB photography or multi-spectral in that the bands involved are narrow and numerous, thus producing a continuous profile. The main characteristic of this technique is the main outcome, i.e. the hyperspectral image representing hundreds of thousands of NIR spectra. A hyperspectral image thus consists of a three dimensional array (cube) of data resulting from collecting the spectral intensity as a function of three different sources of variation (two spatial coordinates, x and y as well as one spectral wavelength coordinate, z) (Figure 1).
As shown in Figure 1 an NIR hyperspectral image carries a large amount of information, as one may extract the full spectrum at each pixel, which can be used for qualitative or quantitative analysis at microscopic level (typical pixel size of 30micron). Conversely, one could analyse the two dimensional (2D) image at a specific wavelength to look for the distribution, homogeneity or heterogeneity of a particular constituent, e.g. oil (absorbing at ca. 2310nm)1 . This is of value when dealing with food quality control issues, especially for the detection and/or identification of contaminants/adulterants or potentially hazardous substances or when the characterisation of the texture or microstructure of products are considered. The possibility of operating at pixel or object level, make this approach the analysis technique of choice when detection or quantification of adulterants is at stake. Analysis of single spectra at pixel level also enables assessment of sample homogeneity or heterogeneity. NIR hyperspectral imaging is thus the ideal technique to use when measuring heterogeneous samples.
To illustrate the application of NIR hyperspectral imaging we refer to two applications: detection and quantification of adulterants in black pepper2 and detection of pre-germinated barley grains3.
Black pepper may be adulterated with millet or buckwheat, both being starch-based adulterants. Based on this chemical consideration, it may be expected that NIR spectroscopy could accurately detect the presence of adulterants in the target food matrix, as demonstrated in Figure 2, where the NIR spectra of black pepper adulterated with millet show clear changes in absorbance values at specific wavelengths, as the level of adulterant increases. Accordingly, since there were samples available for which the level of adulterants was known, it was possible to build prediction models relating the spectral signature to the concentration of unwanted compound. Although such quantification could be in principle carried out also using bulk NIR instrumentation only, the possibility of investigating the problem by means of hyperspectral imaging presents many advantages that will be illustrated.
To build a predictive model for the amount of adulterants, since the reference values refer to the whole sample and not to the individual pixels, one should use the average spectra collected over a representative portion of the images. Since one can tailor the region to be investigated depending on the problem, hyperspectral imaging allows representative sampling even in the presence of high heterogeneity. On the other hand, once the optimal values of the parameters are calculated, the model can be applied to each individual pixel of new (test) images for quantification and distribution studies2.
This idea can be well illustrated by discussing the results obtained in the black pepper example. The Ratio of the standard error of Prediction to the standard Deviation of the validation (RPD) is a parameter often used to show the accuracy of an NIR prediction model, with a value of 3 indicating that the model could be used for quality control. In this case an excellent RPD of 10.37 was obtained. This prediction model could subsequently be used to predict the level of adulterant for each pixel in an image. In Figure 3 the prediction images can be seen with pure black pepper shown as dark blue and the adulterant as dark red. Thus the more pixels indicated as red in the image, the higher the level of adulterant.
Since the samples were analysed with NIR hyperspectral imaging and not conventional NIR spectroscopy, it was also possible to determine the distribution of the adulterant in the sample. Thus, the extent of adulteration can easily be visualised. In Figure 3, the heterogeneous distribution of the adulterant can thus also be seen. Due to the adulterants both being starch-based, differences between the two adulterants are not noticeable in these images. This clear indication of non-homogenous mix of the samples emphasises the importance of analysing larger sampling areas to compensate for this when using conventional NIR spectroscopy. A smaller sample area (indicated as a small square in the figure), typically used for MIR analysis, will thus result in less accurate measurements.
Pre-germination is the untimely germination of mature cereal grains. It is important to maltsters to identify pre-germinated grain. Once barley grains have undergone early germination, it cannot be used to produce malt for beer making. As malting involves rapid germination, the presence of pre-germinated grain in the malt feed stocks will result in decreased malt production. Accordingly, for the brewing industries not to lose precious raw materials and to preserve the quality of the production, there is a stringent need of identifying pre-germinated grains and sorting them from sound ones. In this respect, as illustrated by the second example3, the use of hyperspectral imaging represents a valid and reliable possibility, as it allows obtaining specific information at single grain level.
The whole process of predicting the quality of individual cereal kernels is shown in Figure 4. As there is the lack of a reference method to reliably assess which kernel was pre-germinated or not, at first, principal component analysis was carried out on the training image to define and identify the viable and non-viable grains. Successively, portions of these images were used to build a classification model to predict the quality of future (unknown) kernels, i.e. whether they are sound or pre-germinated. The right side of Figure 4 clearly shows that in a prediction image, sound kernels can clearly be distinguished from pre-germinated ones.
To conclude, NIR hyperspectral imaging systems represent a promising tool to be used to detect and quantify adulterants in food products. Due to their rapidity and versatility, they allow to carry out longitudinal measurements (to acquire images over time to follow the dynamics of a process) and, moreover, being based on hardware which is analogous to that used in digital photography, they do not necessarily require to be in direct contact with the sample. Therefore NIR hyperspectral imaging systems could be used for on-line monitoring during industrial processes or for continuous sorting of products while moving on conveyor belts.
References
- Manley M (2014) Near-infrared spectroscopy and hyperspectral imaging: non-destructive analysis of biological materials. Chemical Society Reviews, 43, 8200-8214 (Tutorial Review.) DOI: 10.1039/C4CS00062E.
- McGoverin CM, September DJF, Geladi P & Manley M (2012) Near infrared (NIR) and mid-infrared (MIR) spectroscopy for the quantification of adulterants in ground black pepper. Journal of Near Infrared Spectroscopy (special issue), 20, 521–528 doi: 10.1255/ jnirs.1008.
- McGoverin C, Engelbrecht P, Geladi P & Manley M (2011) Characterisation of non-viable whole barley, wheat and sorghum grains using near-infrared hyperspectral data and chemometrics, Analytical and Bioanalytical Chemistry, 401(7), 2283-2289. DOI 10.1007/s00216-011-5291-x.
About the authors
Marena Manley completed her undergraduate studies at Stellenbosch University and her postgraduate studies at the Universities of Pretoria and Plymouth in the UK. She is now a Professor at the Department of Food Science, Stellenbosch University where she heads a cereal quality and non-destructive analysis research group. Her research includes studying microstructure of food and agricultural material using NIR spectroscopy, hyperspectral imaging and X-ray micro-computed tomography.
Dr. Federico Marini is a Researcher at the University of Rome ‘La Sapienza’, where he also teaches chemometrics at both the undergraduate and graduate levels. His research interests involve development and application of classification methods, especially in the field of food authentication, nature-inspired methods (artificial neural networks, genetic algorithms, particle swarm optimisation), non-linear modelling and multiway analysis.